Breaking the Boundaries of ACG Art with AI Image Generation
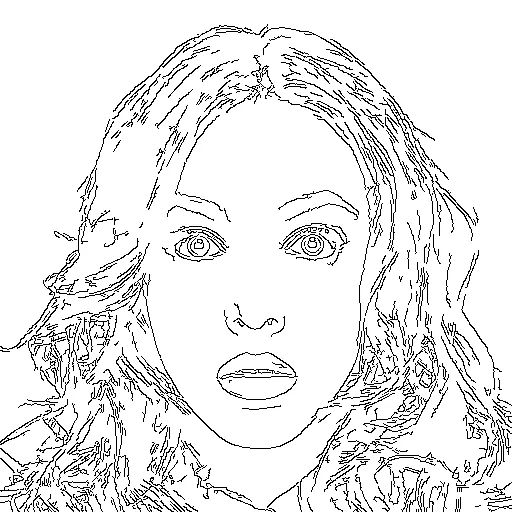
In today's fast-paced world, the ACG (Animation, Game, and VFX) industry demands high-quality visuals that can captivate audiences and provide immersive experiences. Whether it's creating lifelike characters, realistic environments, or breathtaking special effects, the ability to generate visuals that look and feel real is crucial to the success of any project.
Traditionally, creating realistic visuals has been a time-consuming and expensive process that required skilled artists and extensive resources. However, with recent advancements in AI and machine learning, it's now possible to generate high-quality visuals more efficiently and at a lower cost.
AI can be used to create realistic visuals in a number of ways, from generating photorealistic images to enhancing existing images with more detail and depth.
Understanding the basics of AI image generation model
AI drawing, or AI-generated art, is the process of using artificial intelligence algorithms to create images, graphics, and other forms of visual art. The principle behind AI drawing is to use machine learning algorithms to train neural networks on large datasets of images and then use these networks to generate new, original images that are similar in style and content to the images in the training dataset.
To put it simply, we provide the original image and prompt words, generate an intermediate image through the ControlNet model, and then use the Stable Diffusion model to finally generate high-quality images that meet your expectations.
here's a more detailed explanation of how the ControlNet model and Stable Diffusion model work together to generate AI images:
The ControlNet Model:
The ControlNet model is a generative model that works by taking an original image and a set of prompt words or text descriptions as input, and then generating an intermediate image that incorporates the prompts in a realistic and visually pleasing way. The model achieves this by using a combination of convolutional neural networks (CNNs) and attention mechanisms to selectively enhance and modify different parts of the input image.
The CNNs used in the ControlNet model are trained on large datasets of images, and are able to learn complex patterns and relationships in image data. The attention mechanisms, on the other hand, are designed to selectively focus on different regions of the input image based on the prompts or descriptions provided.
To generate an intermediate image using the ControlNet model, the input image is first fed through the CNNs to produce a feature map that captures the visual content of the image. The feature map is then combined with the prompt words or descriptions using the attention mechanisms to create a context vector that reflects the desired modifications or enhancements to the image.
Finally, the context vector is used to generate the intermediate image by applying a series of learned transformations to the feature map. These transformations are designed to selectively modify different regions of the image based on the prompts or descriptions provided, resulting in an intermediate image that incorporates the prompts in a realistic and visually pleasing way.
The Stable Diffusion Model:
The Stable Diffusion model is a generative model that works by taking an intermediate image produced by another generative model, such as the ControlNet model, and gradually refining and enhancing it over multiple steps. The model achieves this by using a diffusion process that adds more noise and randomness to the intermediate image over time, while still preserving the overall structure and visual coherence of the image.
To generate a high-quality image using the Stable Diffusion model, the intermediate image is first fed through a series of diffusion steps that add more noise and randomness to the image at each step. The diffusion process is carefully designed to balance the trade-off between generating high-resolution images and preserving fine details and textures in the image, resulting in a final image that has a high level of detail and complexity.
Once the diffusion process is complete, the final image is produced by applying a series of learned transformations to the noisy image. These transformations are designed to remove the noise and restore the visual coherence of the image, resulting in a high-quality image that is visually consistent and free from artifacts or other visual defects.
By using the ControlNet model to generate intermediate images that incorporate prompt words or descriptions, and then using the Stable Diffusion model to refine and enhance these images, artists and designers can quickly and efficiently generate high-quality visuals that meet their specific needs.
Benefits of Using AI for Image Generation
AI for Image Generation
Artists and designers in the ACG industry can benefit greatly from using AI for image generation. Here are some of the key advantages:
- Enhanced Creativity: AI image generation tools can help artists and designers break through creative blocks and explore new design possibilities. By generating a wide variety of images and designs quickly and easily, AI can inspire new ideas and provide a starting point for further exploration and refinement.
- Time-Saving: Generating high-quality images and designs can be a time-consuming process, but AI-powered tools can significantly reduce the time and effort required. AI can quickly generate a large number of images based on a given set of inputs, allowing artists and designers to focus their time and energy on other aspects of the creative process.
- Cost-Effective: Traditional methods of image generation, such as hiring professional photographers or commissioning artists, can be expensive. AI-powered tools can provide high-quality images at a fraction of the cost, making it a cost-effective solution for businesses and individuals alike.
- Consistency and Accuracy: AI can help ensure consistency and accuracy in image generation, which is especially important for businesses that need to maintain a consistent brand identity across multiple channels and platforms. AI-powered tools can also reduce the risk of errors and inconsistencies that can arise from human error or variability.
Overall, AI-powered image generation tools can provide a range of benefits for artists and designers in the ACG industry. By enhancing creativity, saving time and money, and improving consistency and accuracy, AI can help artists and designers produce high-quality images and designs more efficiently and effectively than ever before.